Enterprise Data - Collection & Insights
- Sachin Tah
- Nov 7, 2021
- 5 min read

IT is all about Information, which is Data, and of course, technology, and technology is a way to perform operations on this data. These operations are what make data very useful and powerful.
Prehistory refers to the period of time with no written records, so whatever we know as history signifies that information is available somewhere in retrievable form for our reference, be it right or wrong.
One of the biggest reasons for humans to achieve incremental growth generation by generation is because of our ability to store, share, transform, and consume data which is also termed as information exchange. This exchange was possible only because of some form of mechanism available for data storage, data distribution, and data consumption. Be it in any form, sculpture, drawings on a cave wall, handwritten transcripts on of metal sheets, information available on paper, books, or in present era data stored on an electronic device which is referred to as digital data.
If you have noticed, data or information is vital right from the very old age, the way we are storing and consuming keeps on changing. Even within the 50-year-old history of IT, data is the key and still takes the centre stage, we are just changing the way it is either stored, consumed, managed, and visualized.
When we talk about the digital transformation journey of an enterprise, the idea is to store and consume all forms of data (information) flowing in and out in a digital format. Some examples would be storing handwritten loan applications digitally, automating claims filing and processing, etc. I don't need to tell the benefits enterprise will achieve by completely going digital. However, to this date, enterprises are struggling and finding it difficult to keep pace with the transformation journey because of multiple reasons.
One is that an enterprise cannot operate in silos, they need to interact with other enterprises, customers, suppliers, and many other entities which may or may not be transferring information digitally. Even if they are, there is always a need for some standard protocols which ensure efficient communications. This is somehow standardized by some industry segments like healthcare, finance, but still, we have a long way to go.
Data generated within an enterprise comes from various sources, be it internal or external, and is also distributed across disparate systems and subsystems. Data is usually consumed by the parent application which generates the data or some other downstream applications and systems. Below is a visual representation of various sources of data available within an enterprise ecosystem
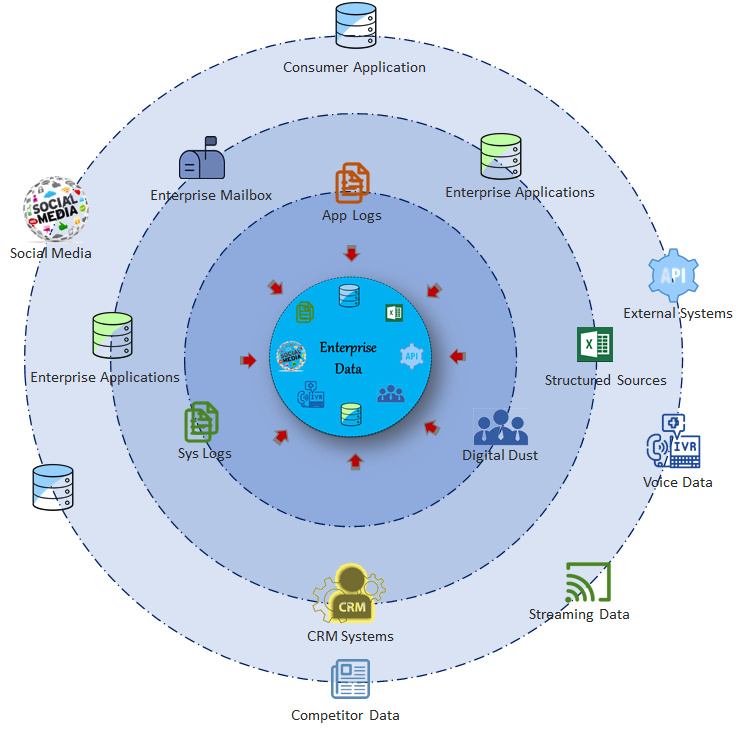
The amount of data generated in a large enterprise is enormous and mind-boggling and I am sure not a single enterprise is making sense of the entire data it holds. At an enterprise level, is there a way or need to have a consolidated view or insight on this data? What all enterprises can learn from this data and can it correct/recorrect their future course?
Enterprises generate data as a part of their day-to-day business operations. Data generated can be further processed to come up with different insights in order to evaluate business performance, competitive analysis and also act upon opportunities for further business growth.
There are four insights you can get from the underlying data your enterprise holds
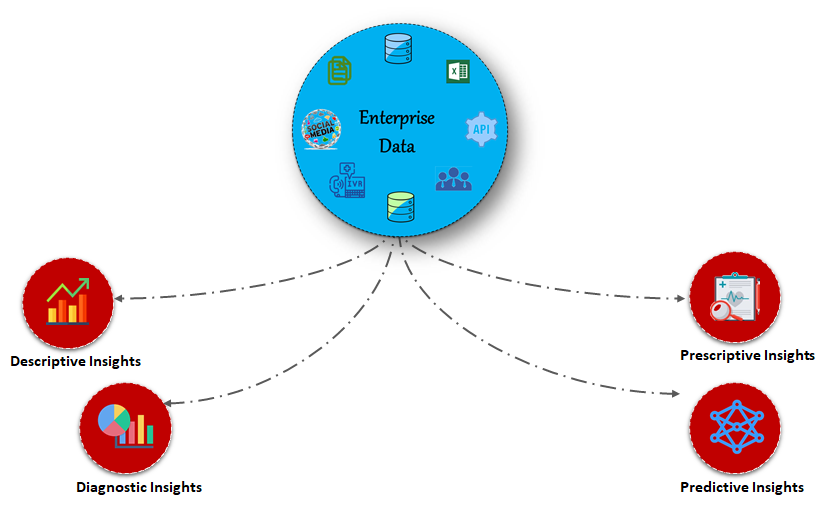
Each of these insights is very useful in order to course-correct the way an enterprise operates, create strategies for the future, and learn from its mistakes.
Descriptive Insights - Historical insights on What happened in the past and used to tell a business or stakeholders how well it is performing.
Diagnostic Insights - Historical insights on Why it happened which is a step further to descriptive analysis. Also referred to as root cause analysis.
Predictive Insights - Historical data collected is fed into ML algorithms to generate predictive models. These help in predictions so as to take appropriate actions.
Prescriptive Insights - Prediction is good, what if you have a prescription on how to deal with a situation? The prescriptive analysis does exactly the same.
The above insights look great and will surely provide the enterprise a competitive edge over its peers, however, there are implementation challenges.
Enterprise data may reside inside their old legacy systems, RDBMS databases, NoSQL, emails, log files, knowledge management systems, system logs, CRM systems, employee digital footprints, streaming sources, and many more. Enterprise-level insights on varied sources of data are not possible. You need to transform the data to come up with insights, however, you never know what level of transformations are required before time, and that too on huge volumes of data. A solution to this problem can be solved by implementing a Data lake.
A Data Lake is a centralized repository that holds huge volumes of data in its raw format. There are many strategies available in order to implement a data lake, conceptually it is the same. Cloud providers like AWS and Azure provide a set of tools and technologies to make life easier.
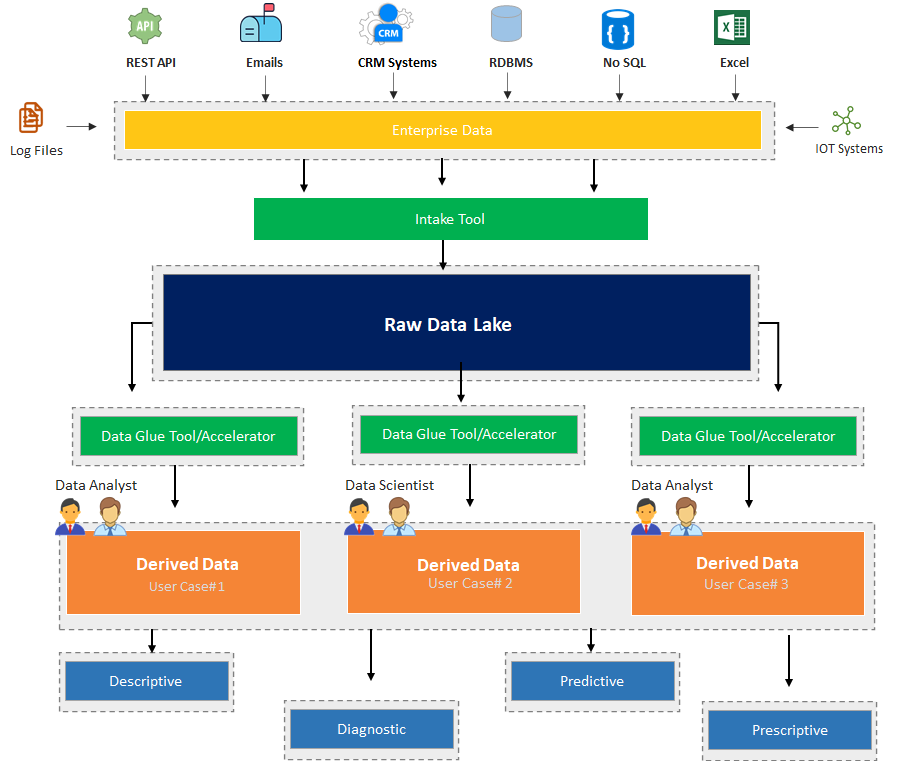
Consumption of data via data lake should be implemented in stages, below are the stages and steps which needs to be performed
Stage #1 - Collect data from various source systems like RDBMS, No SQL, CRMs, Emails, Log Files, Excels, and streaming sources like IoT applications. Store data in as much raw format as possible without performing any level of transformation at this stage, use low-cost storage systems like an on-premise file system, S3, or Blob storage. This raw storage is also referred to as Data Lake. Metadata which is also referred to as a Data catalog should also be created and updated in order to have details of how the data is kept in the data lake.
Stage #2 (Data Derivatives) - Data stored in a lake is pulled on a need basis in order to create derivatives. Create data derivatives depending upon business requirements and business-specific use cases. Again, every use case should have its own dedicated and independent set of data, which means data may be duplicated across derivatives which is fine. Data residing in this tier is aggregated, filtered & transformed depending upon specific business needs. Pull oly data which is needed, For example, forecasting daily sales of a product does not require the purchase history of a product. Data derivatives can be very specific to a use case only. Try to use be low-cost storage systems
Stage #3 (Data Visualisation or Consumption) - Pull data into high-performance storage systems as per the requirements of the end-user. Allow data interactions for analytics by storing the data on high-performance storage or cache systems. Build ML models or use BI reporting to provide a visual representation of the data to the end-user. Data can be consumed for any of the four kinds of analysis mentioned above.
Data derivations and consumptions should be domain-specific and try to solve a business problem. A data pipeline needs to be set up in order to receive continuous feeds of data from the applications generating it.
A typical data journey within an enterprise should look like this
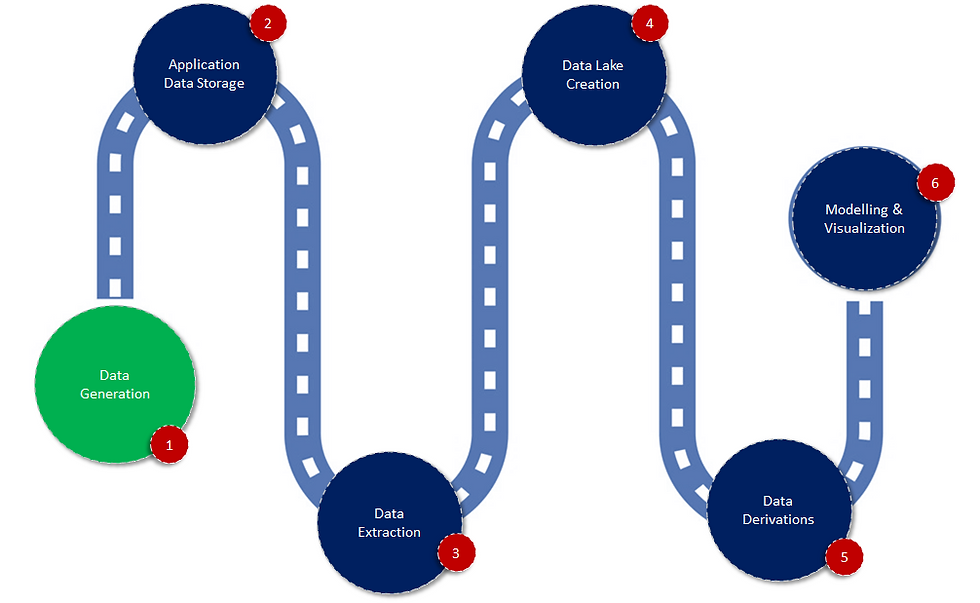
Out-of-the-box tools and technologies are available in order to implement a data lake either on-premise on the cloud. Cloud providers like Azure and AWS come with a handy set of tools that helps in pulling data from any data source to their platforms. Data transformation tools are also available which helps in gluing and transforming data suitable for a use case.
Please do comment and provide feedback on this topic, would be happy to discuss it further. I can be reached directly at sachin.tah@cognizant.com
Happy Reading
Comments